Eshealthtips.com – There are different types of skin lesions. These lesions can be categorized into four categories, each of which has a unique appearance and characteristic. These lesion types are often caused by bacteria. These can affect the face, neck, armpit, buttock, or feet. These lesions are often painful and can rupture, causing puss or clear fluid to drain from the area.
Any Abnormal Changes in the Surface of the Skin
A skin lesion is any abnormal change to the skin’s surface. They may appear as bumps, patches, or lumps, and can vary in size, shape, and color. Some are benign, while others can be serious, even cancerous. Regardless of their cause, many of these skin lesions can be treated.
The most common type of skin cancer is melanoma, and one in five people in the United States will develop it at some point in their lives. Fortunately, early diagnosis can lead to successful treatment and a cure. Malignant melanoma is the most deadly type of skin cancer, but when detected early, it is completely curable.
One of the biggest challenges in skin lesion classification is the high similarity between skin lesions. In recent years, machine learning-based methods have been widely used to improve classification accuracy. Convolutional neural networks, for example, have shown impressive results in identifying skin lesions using a wide range of images.
How to Diagnose Skin Lesions
A patient’s primary care provider may refer them to a dermatologist to diagnose their skin lesions. During the initial evaluation, dermatologists can determine whether the lesions are benign or malignant. If the dermatologist believes that the skin lesion is a cancerous one, they can biopsy it and provide a definitive pathologic diagnosis.
There are three main types of skin lesions. These include nevus and melanoma. Melanoma is the most dangerous type of skin cancer and can spread to other parts of the body. However, it is highly curable if caught in the early stages. Despite the risk associated with melanoma, a diagnosis can be difficult. Human visual factors, such as fatigue and eye fatigue, can make it difficult to accurately diagnose a skin lesion.
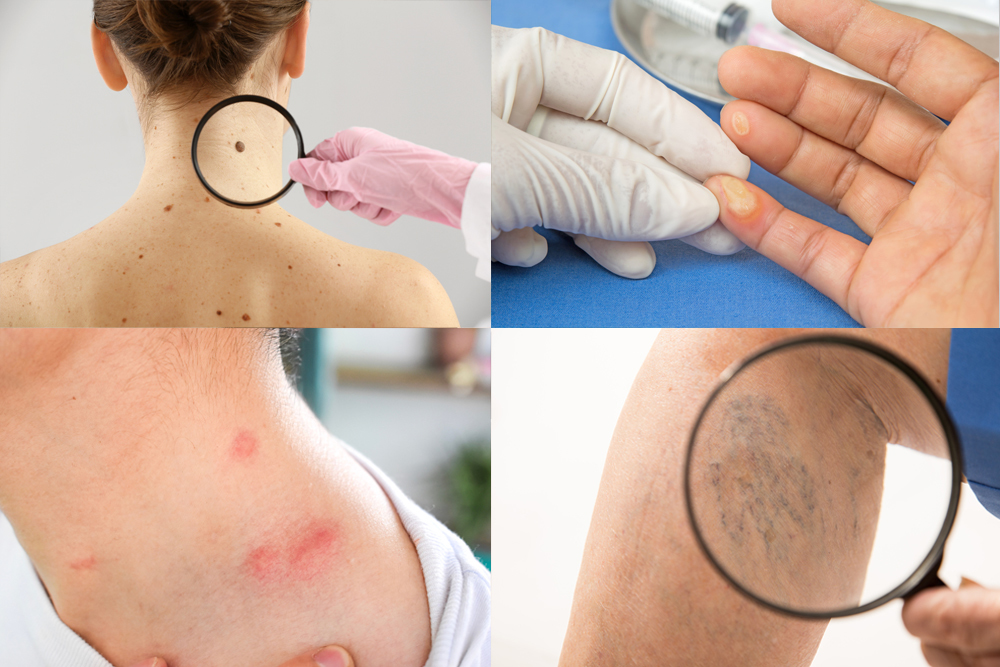
CNNs have shown excellent results in skin lesion classification, but a balanced dataset is necessary to train a good model. However, if the dataset is too imbalanced, the classification performance degrades. However, a two-stage training method – SuperCon – has been successful in detecting skin lesions from images. This method is effective for detecting many types of skin lesions and can improve diagnostic accuracy.
Beware of the Type of Skin Lesions
Skin lesions are common in all areas of the body. Most of them are harmless, but it’s best to see a doctor if a new one appears or a mole has changed. It’s important to be aware of the types of skin lesions because they can be a sign of cancer.
Dermoscopy is a noninvasive imaging technique that helps distinguish between non-malignant and malignant lesions. It involves magnifying and illuminating suspected skin lesions. Dermoscopy is challenging due to the variety of colors, shapes, location, and texture, but it is a powerful tool to help physicians diagnose skin lesions.
Skin lesions are broadly classified into two types: non-melanoma and melanoma. Of the two types, basal cell carcinoma accounts for 75% of melanoma cases and squamous cell carcinoma accounts for 20% of all skin cancers. In addition, skin pigmentation and sun exposure are significant risk factors for skin cancer. Furthermore, immune suppression can lead to the development of certain types of skin cancer.
Reference: